A blog post by Greg McInerny
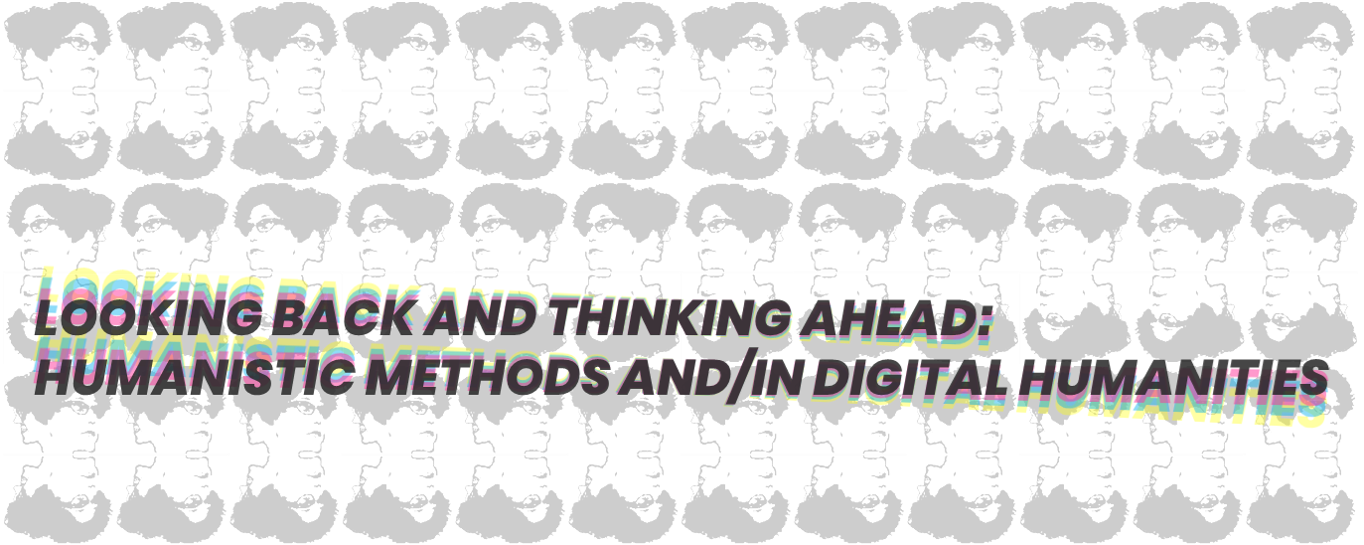
Rather than being a simple ‘power up’ for intellectual work, computers and digital technologies are augmenting academia. It isn’t just a case of buying laptops and servers, collating data, analysing spreadsheets and hiring software developers to join things up. Computational Science is not just science on computers, and Digital Humanities is not solely a digital version of humanities research. Instead, a cascade of new challenges and considerations are precipitating out of our interactions with computation and digital methods.
Recently Johanna Drucker gave a lecture in Cambridge entitled “Looking Back and Thinking Ahead: Humanistic Methods and/in Digital Humanities” (available online here https://www.youtube.com/watch?v=FV23qVqkMas). Through critical reflections on why, what, how and where ‘the digital’ and computation is employed in Digital Humanities research, Drucker explored what issues and questions have now become system critical in academia.
As I left the lecture I wondered whether it had been ‘humanistic’. Were the issues and challenges that Drucker raised specific to the humanities?
1. Critical Pedagogy
Drucker didn’t hold back. Making the most of her experience and the lecture format, Drucker posed a number of challenging perspectives on working with and around machines. She set up provocations that cut to the heart of matters, using examples and arguments that challenge us to say the critiques are not true.
Software can be black boxes. We don’t know what software do.
Perhaps such statements have been said so very many times that the importance of their contention has become diluted? Drucker had an alternative provocation that re-condensed the issue. It is unethical to teach/use software without simultaneously teaching/applying a critical understanding of the methods software invoke.
Targeting the ethics of research and teaching translates a ‘problem’ into a fundamental issue that is harder to ignore. Maybe we have to explain why this ethical issue does not undercut our academic values and principles. For instance, if academia is based on rigorous analysis and principled arguments, then our software use should be based on a critical understanding of the methods they employ and the sensitivities of those methods. Furthermore, if we make an intellectual claim based on software then its validity is, at least in part, reliant on us having a critical, ethical relationship with the software that enables our intellectual claims.
A new critical pedagogy, or furthering the reach of existing pedagogies, would assist in addressing the issues lurking in uncritical use of software. But don’t we need more? What constitutes ethical software use? Coding skills sufficient? How about a theoretical understanding of methods? Or experience and expertise informed through practice? How do we attain and prove we have sufficient license to use software as methods?
These are open questions about how academia uses and relies upon software. Inviting, or even nurturing, a blindness to methods and the forms of argumentation invoked in software could take us toward precarious positions. If we don’t know the principles operating within software then what should others make of our intellectual claims? Do we expect different levels of scholarly engagement when it comes to software?
2. Standardised research.
Drucker really does not hold back. As Drucker articulated it, research is becoming standardised by popular software tools (such as Gephi, Leaflet, Cytoscape and Omeka). But isn’t this what we wanted software for? I.e. to provide standardised, tested tools for particular jobs.
The provocation proposed by Drucker is that software may be stifling our creativity and we are seeing research through reduced possibilities. Our research may become defined by that software and in the case of visualisation tools, such as Gephi, our research can literally start to look the same.
This is not necessarily a critique directed towards the software projects. Perhaps this critique is one of the best demonstrations of the software doing its job? The critique points towards the researchers and academia who are using them, or using them in a particular way. Do we have the strategies to get out of the gravitational pull of software when we need to?
On one hand we want impactful tools that assist our work, reducing the need to invest in our own methodological and technical skills. We can then invest in the rest of our research endeavours and make use of methods that would not otherwise be tractable.
But what is on the other hand? Critique? General discussions on how to develop/use/support/sustain digital infrastructures? New kinds of hybrid jobs in academia? Maybe we should start with deeper investigation of what factors create an apparent pull towards certain software and how popularity becomes an issue?
Drucker pushed back on the response of humanities subjects which “critique but don’t create”. For instance, Digital Humanities is closely related to subjects involved with in-depth, strong critiques of technologies – such as software studies and media theory. Drucker wants the critiques to be deeper and have a productive feedback with tools, infrastructure and code.
That is not to say that Drucker bestows privilege onto disciplines that “create and don’t critique”. If there are two cultures neither is addressing the real issues. We need a third culture.
Underneath these provocations was a call for the humanities to take ownership of their digital infrastructure and the issues it creates. One outcome of greater ownership of our digital infrastructures would be to reduce our blind attraction to “cheap effects” which software can afford us.
Drucker is concerned that software could start to own our research questions. Research questions become articulated through software’s functionality and the answers software are producing are then be articulated through the functionality of other software.
3. Data Models
A number of times Drucker mentioned the importance of data models which define the relationship between a phenomena and data of that phenomena. One example Drucker used was “Rothko Viz” (http://ereyes.net/rothkoviz/) where an analysis of the colours used in images of Rothko’s paintings produced a simple plotting of colour relationships.
The result is an analysis of the data and not necessarily an analysis of the phenomena. The result relates to the images and not the paintings.
Without knowledge of how the data (the images) were collected – the lighting, scanning equipment, filtering, post processing, scale, distance … – then whatever we interpret from the data is contingent on an assumption that the data model is insignificant. It is however the data model that articulates these differences between phenomena and the data.
This is not necessarily an issue if the data model is invariant. However, if the data model varies across data sets, then biases in the data can become errors in our inferences, and these errors become mistakes.
Critiquing the Rothko Viz example may have been a tad strict. In my estimation it seems to be a personal project that experiments with methods, rather than necessarily laying substantial claims to new knowledge. Though it was a good example to use.
For Drucker, the key point is that data models and/or its implicit assumptions are rarely recognised or surfaced. This observation may well be more broadly applicable.
In Science the effect of a data model may be known as observation and measurement errors in the data, which also include sampling errors (for example, not all of Rothko’s paintings are in the National Gallery of Art website from which the images were sourced). And data also contains ‘process errors’ which are generated by the mechanisms and structure through which the phenomena is manifested. A simple example being fluctuations, instabilities and non-uniform patterns in the systems that we are trying to understand, such as traffic jams, the spread of flu across a region or the stripes of a zebra. In the case of Rothko Viz, we are assuming that there is an equivalence and stability in how Rothko-ism is realised in these images.
Perhaps we can all do more to explain what our data is, and what we think it is. Doing more requires that concepts and terminology surrounding data models (or observation and process errors) become an everyday component of our discussions and investigations.
4. Another aspect of critical pedagogy
Given the principles being discussed, it seemed that the topics were becoming less and less about humanities specifically, and more and more about contemporary research methods.
Drucker proposed that a basic prerequisite for Digital Humanities training should be sampling and modelling (as well as the production and publishing of online interfaces). An understanding of sampling and modelling are absolutely fundamental to taking ownership of data and the inferences that are made from data.
This is something that is crucial in sciences, but something I have heard less of in my relatively small experiences with Digital Humanities and related disciplines.
I lost my concentration at this point of the lecture. I was contemplating where/how, under Drucker’s proposal, statistics enter into digital humanities research and what the response would be. My take from this section is that Drucker was questioning our ownership of our subjects if we don’t ‘own’ this basic methodological knowledge. How can we reflect, critique and be reflexive if this isn’t understood?
Paraphrasing Drucker ‘If you don’t know how to make something then you don’t know how it works’. And that includes the making of data sets.
We have no desire to compromise ourselves, and future research, by not placing these issues into our (expanding) critical pedagogy. So let’s put it in.
5. “Boutique Projects” versus “Contributions to a research infrastructure”
Drucker also made some challenges to what we fund, support and sustain in research.
“Boutique projects” are more likely to provide rewards in the short term, such as publications, reputations and invitations. Boutique projects demonstrate possibilities, stimulate thinking and inspire.
However, a production line of neat/cute projects will not produce a robust digital infrastructure. The success of boutique projects may stimulate others to emulate them, producing more boutique projects that never contribute to the technical infrastructure from which they are benefitting.
The longer term view has to consider the legacy of Digital Humanities projects by doing the work that can seem boring, onerous, too technical and that may seem to slow research down. It is obvious why this work is so easily discounted against and postponed in favour of short term gains. Especially if we don’t cement a critical pedagogy into our work. Indeed, without a critical pedagogy boutique projects may be favoured.
Drucker doubled down on this by saying “every digital project is on life support” and that many should have the “plug pulled”. Without contributing to future research work, how does a project prove its worth?
Where do we go from here?
I was disappointed walking into the lecture. I had bumped into a former CIM student who told me Drucker had co-organised a visualisation workshop earlier that day. I thought I’d missed the best part of her visit to Cambridge and sat at the back.
But, I left feeling invigorated and challenged as the lecture had provided new fuel and motivation. Surprisingly, Drucker had made me think about science. A lot.
In many ways there was nothing new in Drucker’s talk. Computational Science has been discussing the same or similar issues in the time that I have been around. What Drucker provided was some alternative articulations, new twists on long standing issues.
The upshot, for me, is that being computational is not just a matter of using computation, being digital is not just inserting digital methods. It could be. But then it may never be anything more. Remaining scholarly, innovative and critical amongst the opportunities of the digital and computation requires that we engage with the epistemological, behavioural, cultural, social, political and design issues of this research. Can we actually manage our responses to its affordances and ensure that our research is enriched rather than impoverished? If we are teaching through software then are we teaching actually digital humanities or computational science?
I very much share some of the priorities that were suggested in Drucker’s lecture. A focus on user interfaces, interoperability through simplicity (Drucker’s “poor media”), and strategies that ensure we can construct and sustain multi-/inter-disciplinary teams despite the institutional and cultural challenges of doing so.
A re-articulation of these issues does not necessarily come with a larger strategy of how to address them. The symptoms are not the cause. But the way they we articulate and communicate these issues can help us to develop that strategy.
Software can de-skill, it can distance and disguise. Boutique projects can divert resources and divert ambitions. Errors can be duplicated without surfacing data models.
“Thinking ahead” has become a necessity. If we think ahead when we teach, publish data, develop software and write project proposals then we can exert a larger, more productive influence on our digital/computational disciplines. Perhaps that is where we start taking greater ownership? But we need a substantial discussion on what that ownership should look like. And how do we produce and sustain an environment where we can take ownership?
One of Drucker’s critiques of visualisation is that evidence is not separable from argument. Perhaps the challenges that Drucker is outlining for Digital Humanities and those of Computational Sciences are essentially the same. Is research separable from method? Is method separable from software? Should they be separable? If so, how?
Lines of sight
In the closing of her lecture Drucker complimented Cambridge itself, by suggesting this assemblage of gown and town serves as an inspiration for progressing Digital Humanities.
“One of things I notice as I walk around is the number of frames, and framings, there are here… Every view and sightline has frames within frames within frames.
Those frames give you a point of reference in which you are situated but also seeing.
There is a spatial articulation of experience… that has a profound effect on the cognitive processing of the environment.
And this we have not begun to explore adequately within the digital environment.
We have used the simplest solutions, flat screen spaces, very unthought through knowledge design, and replicated standard platforms and protocols.
I would say learn from Cambridge… look at this world and how it works, it could be terrific point of departure for thinking about how knowledge design and cultural materials could be articulated.”
We need to think around how we have framed digital and computational disciplines. There is a diversity of interdisciplinary challenges for which a variety of forms of interdisciplinarity could be applied.
Issues of access, participation, diversity and privilege should also be reflected if we take ‘Cambridge’ as inspiration.
Such issues were introduced by Drucker too during her lecture, in other ways, and need to be a key consideration when we are “thinking ahead”.
It is clear that the way forward isn’t solely humanist. We have something larger on our hands that also involves technological, digital and computational issues, but examined though intersecting cultural, social, epistemological, methodological, practical, visual, architectural and geographical frames.
I hesitate to say this represents an ‘interdisciplinary’ set of issues/challenges/opportunities. It does. But there is a need for deeper, more detailed and pluralistic articulations of these issues/challenge/opportunities than one word can achieve.